Real-Time Statistical Process Control (SPC) Software for Manufacturing
- Continuously improve and optimize manufacturing processes with SPC software from Advantive.
- Get the strategic insight you need to make data-driven decisions that improve product quality, decrease costs, and reduce waste.
- Quickly detect and prevent problems in the manufacturing process before they arise.
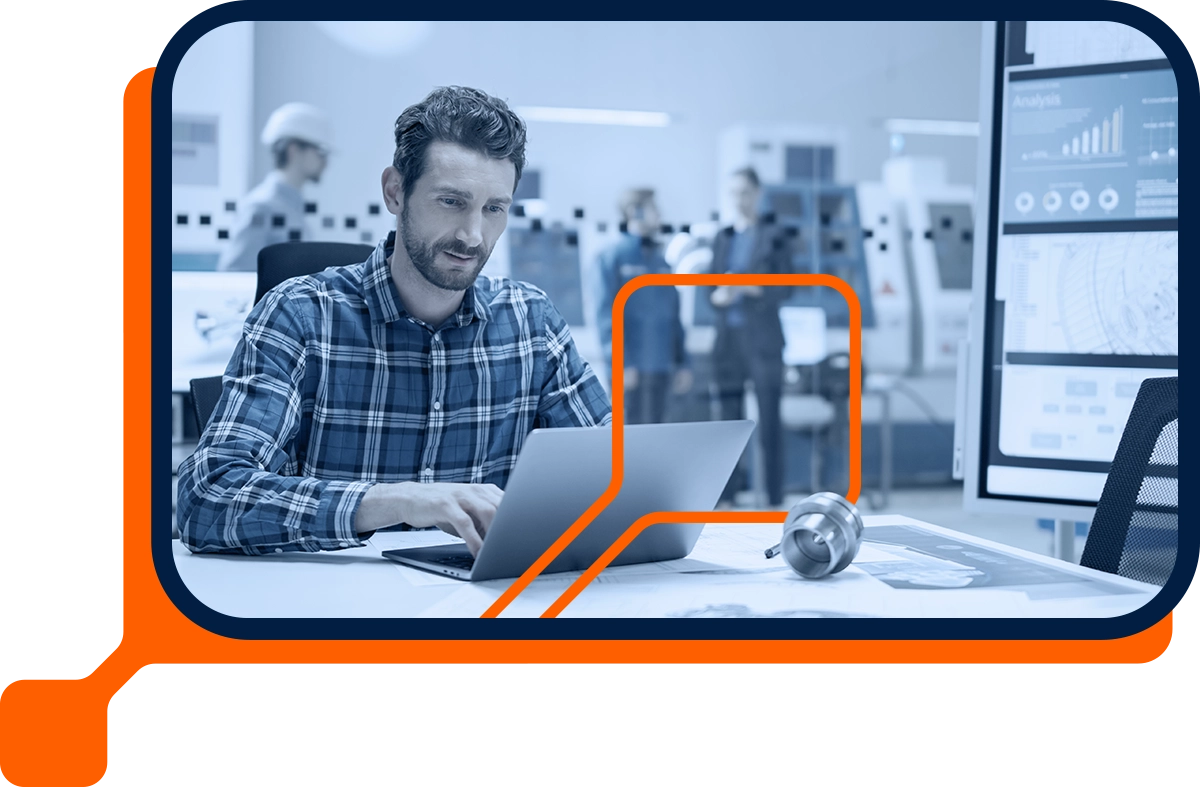

Control Process Inputs on the Production Floor
Take the guesswork out of quality control with statistical process control (SPC) software. Advantive’s award-winning SPC software delivers unparalleled visibility and intelligence to help plant managers understand and control variability in manufacturing. Developed by experienced statisticians, quality professionals, and Six Sigma-certified experts, our real-time SPC solutions include the measurement and monitoring tools you need to minimize production costs, optimize product quality, and significantly reduce risk, defects, and inconsistencies.
Scalable SPC Software to Drive Better Quality Outcomes
With real-time quality data from across the enterprise, our proven SPC software for manufacturing provide simple, intuitive shop floor operator interfaces and powerful analysis capabilities for quality professionals and managers. By aligning operations, process control, supply and demand data, and front-office applications, Advantive’s solutions turn quality risk into a competitive advantage.
For manufacturers who use SPC or are engaged in continuous process improvement activities, SPC control charts are powerful tools for assessing and improving process quality. Control charts provide immediate, real-time indications of significant changes in manufacturing processes that warrant a root-cause analysis or other investigation. From standard control chart options for high-speed production to managing short runs and large numbers of part features, our SPC software offers a huge variety of configurable control charting options to help manage your biggest challenges.
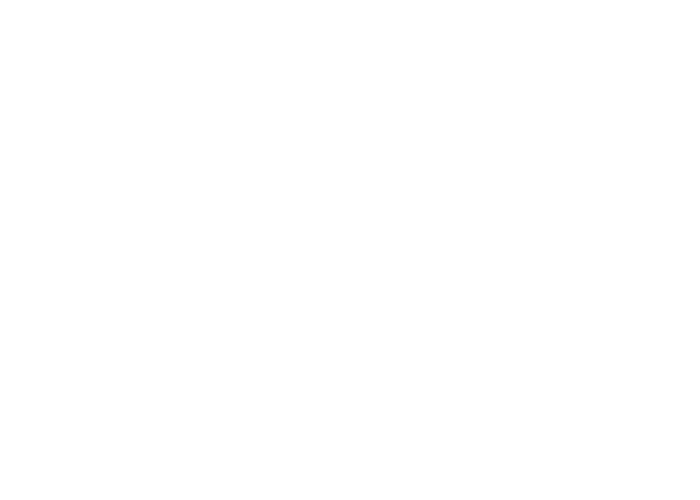
Interactive Guide
With the right Statistical Process Control (SPC) software, your data can provide actionable information to improve performance, reduce production costs, optimize product quality, and increase consistency across your manufacturing operations. Learn more by exploring our interactive online guide to SPC software and quality management.
What Our Customers Are Saying
Find out how our customers are solving plant floor problems, minimizing production costs, and maximizing customer value with best-of-breed SPC software from Advantive.
What to Expect
- Free 20-minute call with a product expert
- Live demo tailored to your industry requirements
- Discover what products best fit your needs
- No games, gimmicks, or high-pressure sales pitch