Introduction to ProFicient
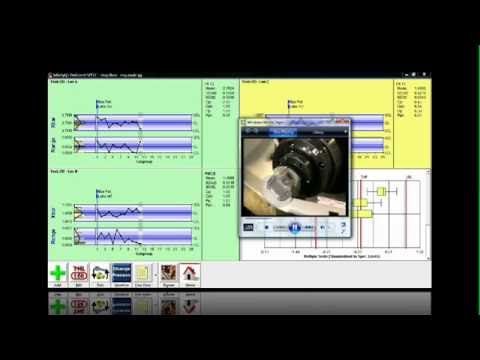
Welcome to ProFicient, the most trusted and powerful real-time quality control and SPC software on the market, and the flagship of the InfinityQS International line of products.
Use the button below to view the resource.
View Resource